Multi-model
·
7 min
Why backplain?
Backplain provides users with choice so that users do not need to rely on any single LLM, which for the organization, translates into no vendor lock-in.

3 Reasons...
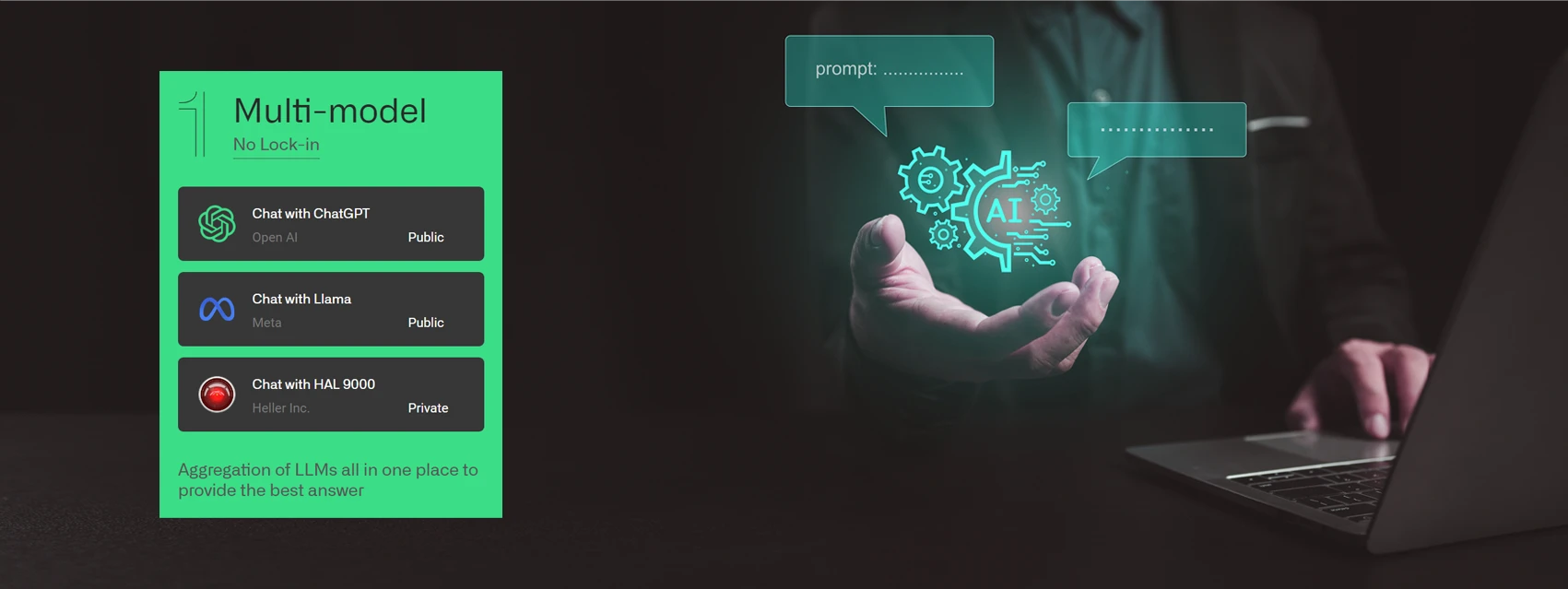
What if your LLM of choice is temporarily unavailable (as ChatGPT was December this year), prohibited due to regulations (that continue to evolve due to privacy and bias concerns), or found to be untrustworthy and/or unreliable (due to poor customer assurance policies or perhaps a model that “collapses” when the data on which they are based cannot be identified as having been machine-generated)?
Backplain provides users with choice so that users do not need to rely on any single LLM, which for the organization, translates into no vendor lock-in.
Backplain supports any combination of public, private, proprietary, and open source LLMs deployed either on-premises, in private cloud, or public cloud as organizational context dictates.
What if you don’t know which LLM(s) are the best fit and most accurate for your organization’s particular use cases.
Backplain allows users to perform multi-model comparison and experimentation from a single, simple, user interface. Users can easily see which models return the “best answer” using their own critical thinking.
What about inevitable LLM proliferation? The next “winner”?
Backplain enables deployment, orchestration, and replacement of LLMs to match organization needs; everything is changing with the introduction of Generative AI so you will need to quickly tap into rapidly moving advancements or be left behind.
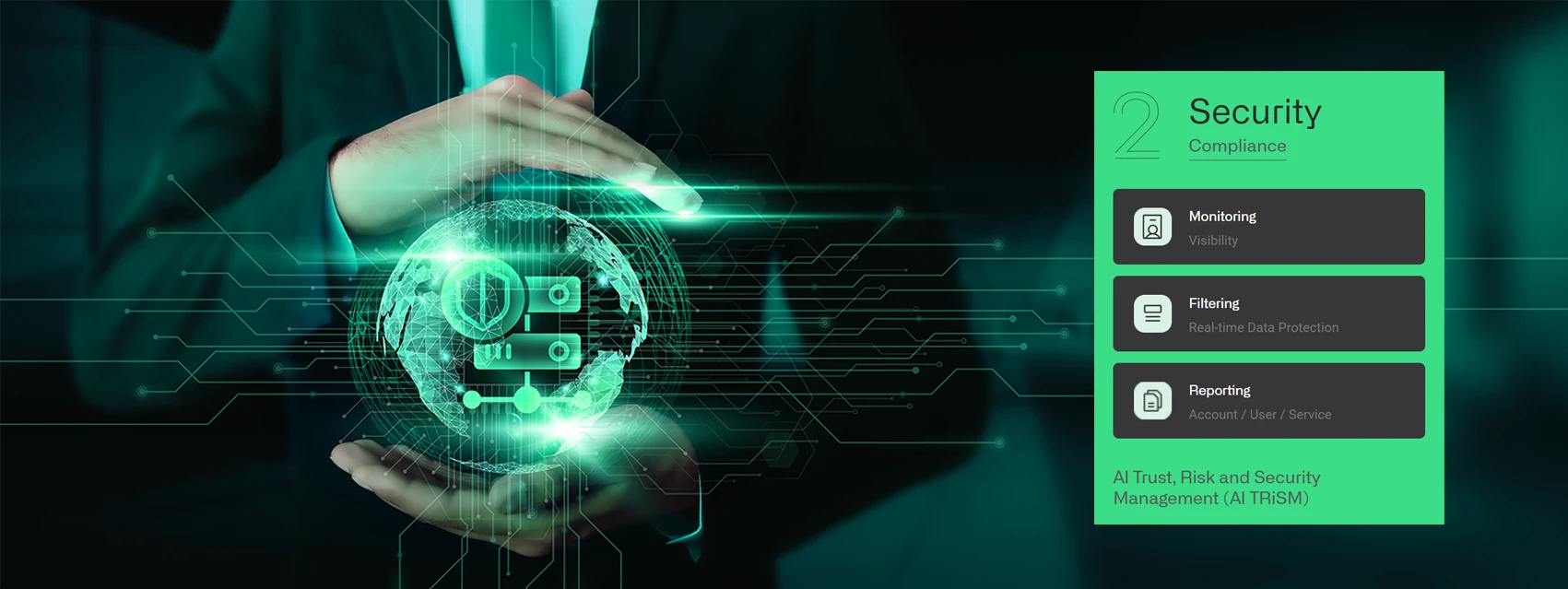
What if IT has taken the position that for you (in marketing, HR, legal, development), the use of Generative AI tools such as ChatGPT are not allowed? Do you use them anyway?
Backplain provides the enterprise guardrails that IT needs to support the safe and secure use of ChatGPT, etc., ending the potential for unsanctioned Shadow AI. With IT as an enabler not a blocker, departments can more easily overcome C-suite security objections to their use of Generative AI.
Backplain supports Trust, Risk, and Security Management (TRiSM) with content anomaly detection, proactive data protection, and risk controls for both inputs and outputs.
When your employees use Generative AI could your Intellectual Property (IP), personal identification information (PII), personal health information (PHI), and other proprietary information be being leaked? More critical could be the inclusion of, for example, API keys inadvertently included in a developer coding request that would potentially open up the entire organization to a security breach!
Backplain filters and masks (as you dictate) to ensure only the information you want is allowed outside of your control.
Backplain provides reportable, auditable, model use so organizations can feel confident they know how Generative AI is being used and how much it costs! As the adage goes “If you can't measure it, you can't manage it.”
On the other side of the coin can you guarantee you are not infringing on someone else’s IP or copyright, hence opening you to potentially costly legal liability?
Backplain will include "compliance checks" by incorporating Human-in-the-Loop (HTIL) as part of publishing workflows, providing the ability to validate Generative AI output - if it was created using Generative AI then it can be flagged for scrutiny.
Do you trust the corporate giants providing the LLMs to police themselves? Who watches the watcher?
Backplain acts as an organization’s own independent AI watchdog.
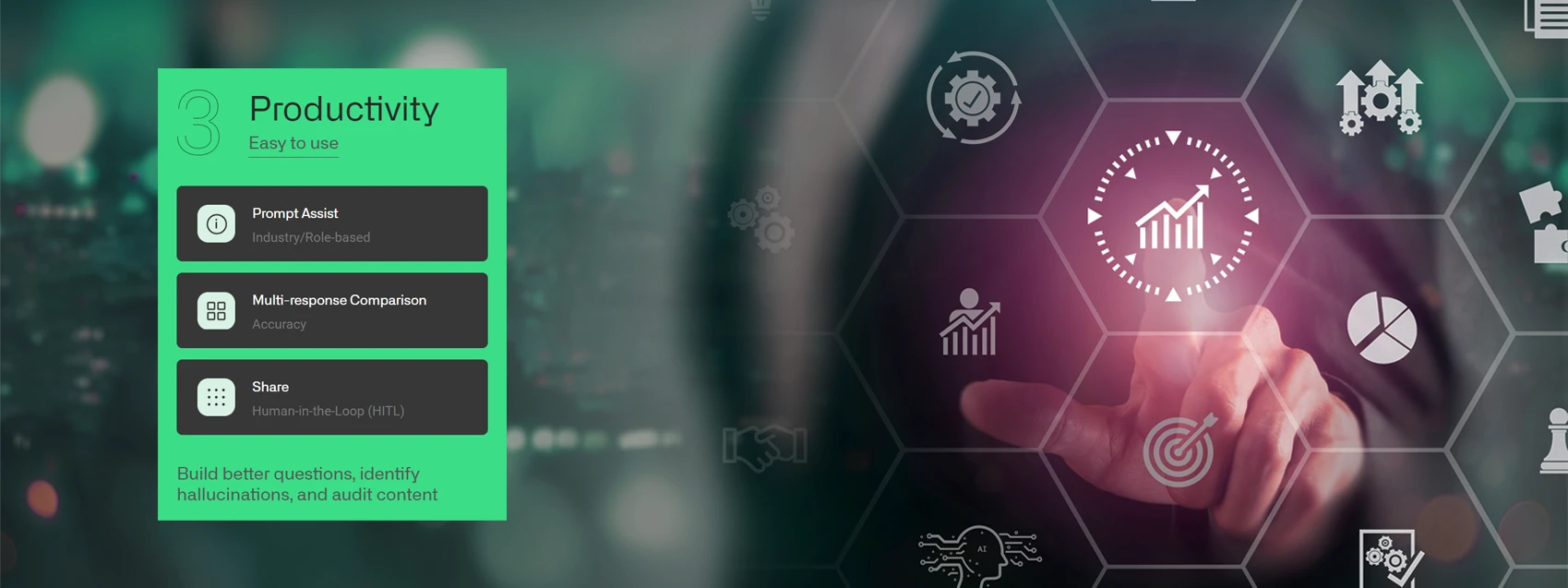
“Gen AI is not interested in the truth – it is only interested in the probability of which word should come next.” This means that some Gen AI-generated answers look credible even when they’re incorrect. Can you afford for the answers to be inaccurate or completely fictitious?
Backplain supports query input into multiple LLMs simultaneously and then subsequent comparison of the result outputs. This is often the simplest method for the user to self-identify potential hallucinations aka inaccuracies and fabrications.
Backplain users can rate query success so the system itself can learn what makes a good query vs. a bad query (which in turn impacts query improvement – below).
Accurate yes, but is the answer adequate? Are you creating poorly constructed queries?
Backplain is continually developing methods to help you improve your queries through what is known as prompt engineering, and the sharing of query construction, success, failure, and improvement in the form of Prompt Notebooks - another example of the value in having Human-in-the-Loop (HTIL) interactions. For many organizations this can offset potential skills shortfalls.
Backplain can include citation and source where possible.
Is there a lack of contextual relevance relative to your organization? Is the information out-of-date or too imprecise?
Backplain, through our sister company Epik System Data & AI Practice, can provide the services to build and implement Retrieval-Augmented Generation (RAG). By building a vector database from your own data, relevant documents are retrieved using a retrieval model, and then used to augment the input context, providing the generation LLM with additional information. The selected LLM is then used to generate output, conditioned on both the input context and the retrieved documents.
Is Generative AI working on poor data or as they say, “garbage in, garbage out”?
Backplain, again through our sister company Epik Systems Data & AI Practice, can work with you on data preparation for use either through RAG, or for your own Fine-tuned Private LLM. Models are good, data is better.
Try backplain for yourself!
